Autogeneration of a semantic layer - the key for AI/BI
There's a lot of talk about how AI can allow BI-like functionality given a robust semantic layer. That's great, but how do you get to a robust semantic layer?
A couple of days ago, Tristan Handy, CEO of dbt Labs, shared his view of how BI will change with the advent of AI. In it, he discusses how the whole paradigm of BI will shift with the help of AI. Given a robust semantic layer, “AI will far outstrip BI for exploratory data analysis (EDA)” he states.
I fully agree with that statement - as we feed better data, data context and business context into AI, we will be able to do more without needing to resort to BI. Analysts will be able to do exploratory analysis, where they are exploring the data and beginning to comprise their initial understanding of it, without needing to use BI.
Tristan is not alone. We are seeing similar content from many other industry leaders, including Databricks who state: “Picture this: Your backlog of BI dashboard requests dwindles to a fraction. And your business users are interacting with the data directly, getting insights instantly.”.
At the same time, I’ve previously stated that data chatbots are still not yet a reality, and almost no one has AI deployed in production (yet).
How do you reconcile all this conflicting information?
The speed of AI development is insane
We’re all seeing this - every week there’s news of one, or a few, new capabilities that AI didn’t have just a few weeks ago. Whether it’s converting your photo into a Ghibli cartoon, or creating robust video with retention, or models with reasoning, the speed of innovation is insane. Things that we thought weren’t possible just a few months ago are now possible.
That means that capabilities like Text2SQL, which were full of hallucinations in 2024, are starting to become more possible.
On top of this, the AI industry is beginning to look for standards that will help components communicate better with one another. The hot term of March/April 2025 is MCP (Model Context Protocol). It’s a protocol promoted by Anthropic, and now accepted by OpenAI (and soon Google?), and is meant to allow the consumers of content for AI purposes to have a more standardized access to the producers of such content. Various software vendors are racing to implement MCP interfaces to allow AI tools to consume content from them, and align with the new industry standard.
Will MCP really become a standard? Who knows… but the effort to figure out a way to solve this challenge is very much welcome and valued by the entire industry.
Semantics are still a major hurdle
There are many different technologies for articulating/mapping/defining the semantics of an organization’s data. You have dbt Semantic Layer, Cube, Snowflake’s semantic model and dozens of others. If you are successful at defining your semantic layer, you can get a result that looks like this:
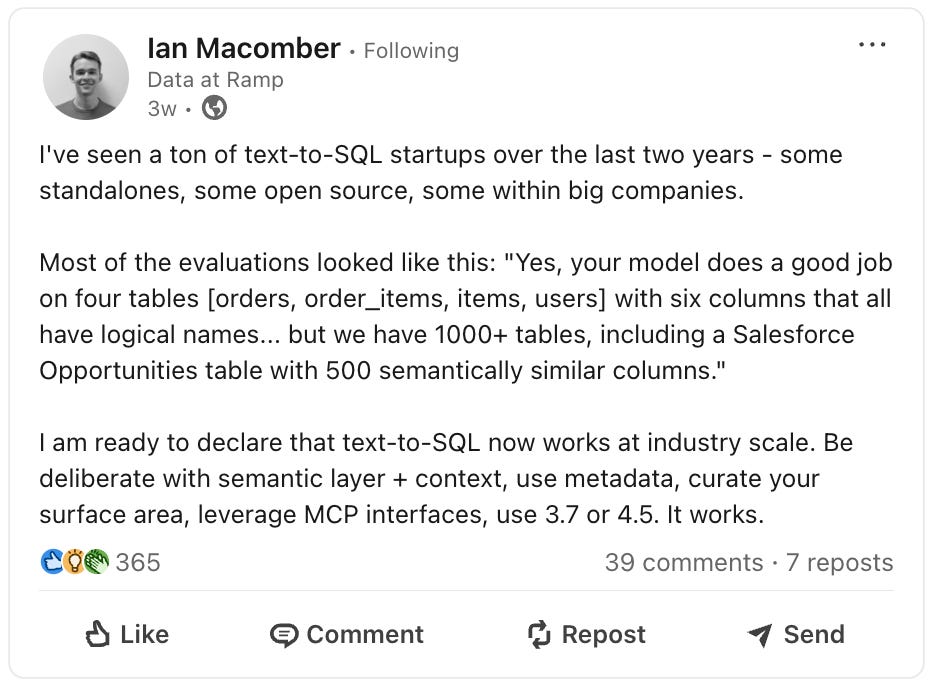
But, what we’re hearing from data leaders is that defining the semantic layer manually is an enormous amount of work, and specifically the kind of work that people hate doing. We’ve recently met with an organization that spent all of 2024 building their semantic layer (Snowflake + dbt) and are now roughly 10% there.
You can compare this to the Waymo journey in San Francisco. We had Waymo’s driving around the streets of San Francisco for several years, with human drivers inside, and humans outside tagging photos, before we got to the point where Waymo can drive on its own and make the trip “unremarkable”.
So, if AI/BI is around the corner, assuming we have a robust semantic layer, and building a robust semantic layer manually is an arduous process, what is the next step?
Building an AI that can generate the semantic layer on its own, of course.
The team at Solid is building this. We’re happy to show you what we’ve got, and where we’re going, hit us up.