(Almost) no AI in production
Last week our team participated in the Gartner D&A Summit in Florida. They came back with many insights - a few of them around the adoption of AI in the enterprise.
“AI is a lot of things. People bucket ML, GenAI and other forms of AI into AI. We’ve been doing ML for many years successfully… so no issue there. It’s GenAI that’s still very tricky”, a Chief Data Officer of a well known equipment manufacturer in the US told me over coffee at the Gartner conference. “The trouble is, our CEO keeps saying he wants all employees to use AI, but it’s not clear how or where.”
He’s not alone. In a recent survey of CDOs conducted by Monte Carlo, 100% of the CDOs said they are being pressured from the top to implement AI. “Your CEO goes to Davos, hears about the magic of AI, and comes back wanting some of that magic for his company”, said Nate Suda, a Gartner analyst at one of the conference’s best talks.
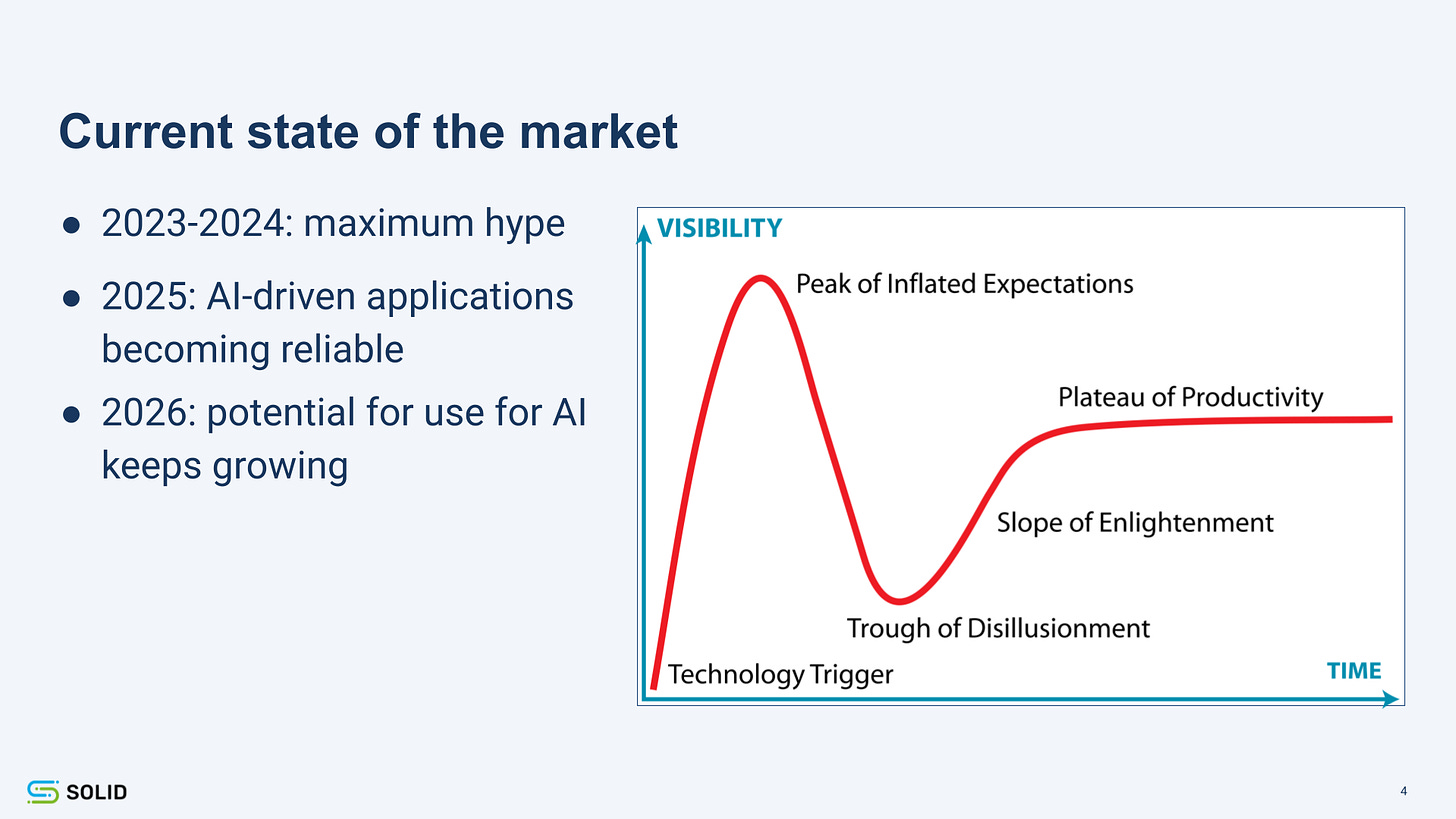
Suda continued - “There are three forms of benefits AI can deliver. A ‘Defend’ approach, which attempts to provide some efficiency gains using the likes of Copilot. An ‘Extend’ approach, where specific workflows and processes get accelerated and improved through AI. And, an ‘Upend’ approach - where an industry is reinvented through AI.”. Nate made the case that “Extend” is where you’ll see the most ROI, the soonest.
Ticking the box with Copilot
Many organizations have opted to start with the first step - buy Copilot (or get it for free in a Microsoft ELA). Some are even forced to buy a Copilot-like tool through price increases. The trouble is that if you just buy Copilot and throw it at your employees, they usually don’t know what to do with it.
When you present someone with a blank, white box, and tell them they can use it to save time and increase productivity, they might not know what to do. So, while Microsoft initially touted impressive numbers, other research over the past year and a half showed a more nuanced and narrow impact, or that the impact is largely limited to saving 14 minutes per day.
14 minutes per day, in an 8 hour work day, is roughly 3%. It’s also the length of a coffee break. Not a huge impact. AI was supposed to make a much larger impact than that (if we want to justify $1 trillion of investment).
Still - it gets your CEO off your back, no?
Getting real AI benefit - drastically improving existing processes
The real ROI, Nate Suda stipulates, comes from focusing on an existing process in your organization and making a real impact on it. Something to the tune of 20%-30% improvement. Think of a process that happens thousands of times, across hundreds of employees, every single day. By building a use-case-specific AI solution, you can make a meaningful impact.
One thing Suda cautions is: make sure you have baseline for the metrics you’re looking to improve in that process… otherwise you’ll have a hard time measuring the true impact. (more on that in another post)
Well… with this use-case focus poses quite a large challenge. You see, taking a generic LLM (ChatGPT, Claude, whatever), and throwing it directly at that specific use-case you picked is likely to fail miserably. Enterprises around the globe have been discovering that in 2024.
Instead, you need to do quite a bit of work to help the LLM provide true value in that use case. LLMs by themselves are not enough. You’ll need to tie in RAGs, provide context, mix in the correct knowledge and construct the fitting user interface for the use case. Or, you can buy an off-the-shelf software from a vendor that has done all that for you (what is now known as Vertical AI companies).
As I was conversing with people at the Gartner conference, I heard the same thing again and again - almost no one has their home-grown AI-for-specific-use-case solution in production. You heard about a lot of people building things, but almost no evidence of production rollout (other than Lippert).
Quite depressing.
So are we screwed? Will AI not live up to the hype?
I’m a very strong believer that it will. I use AI dozens of times a day personally, and it’s solving real problems for me. We’re also building an AI solution for analysts and business stakeholders, and see first-hand through our users that it makes a real impact (accelerating certain tasks by 30% and truly shortening time to insight).
What I think we’re all coming to the realization of, is that AI is actually really hard to get right. You can’t just throw an LLM at a use-case and expect high-accuracy, high-value results. You need to structure a multi-layered engine, that contains multiple LLMs, multiple RAGs, automated feedback/testing mechanisms, deep usage analytics and much more to get it right. And don’t forget - you need to get the user interface right.
Most enterprises will find out that building this by themselves sounds nice, and fun, but it’s like shoveling through mud. It also ends up being really expensive, much more than buying a solution that is built for the use case (with a support contract, all the required integrations, and so forth).
“That’s all very nice, but we’re in the watch-and-wait mode right now”, told me an enterprise architect at the conference. He works for a company with 500,000 employees, and getting such initiatives off the ground is slow and costly for them. His company is not the kind to jump into adventures.
So, just like the Crossing the Chasm book describes, you’ll have your early adopters, and you’ll have your laggards. Early adopters will see real impact in specific use cases soon, by partnering with vendors who are highly focused on those use cases. Laggards will reduce their risk, but also push out the benefits reaped from AI further out into the future.
Which bucket does your organization fall into?